For example, multiple restingstate networks can be revealed from a single rsfMRI study without the need to administer one or more prescribed behavioral tasks, typically by measuring BOLD signal correlations relative to a "seed" region of interest, or by using multivariate component models to identify networks based on statistical criteriaCalculate group average of Fisher z scores 4Perform two sample ttest of group averagesRestingState fMRI rsfMRI is a method aimed at examining intrinsic networks in the brain while no task is performed (rest);

Functional Connectomics From Resting State Fmri Trends In Cognitive Sciences
Seed region fmri
Seed region fmri-The middle panel shows a timeseries extracted from a seed region The bottom panel shows the interaction regressor, created by multiplying the above regressors Note how the 1's, when multiplied by the seed timeseries, invert the sign of the timeseries if the timeseries is going down, in the interaction regressor it will go upFunctional magnetic resonance imaging (fMRI) has become a powerful tool to study brain dynamics with relatively fine spatial resolution One popular paradigm, given the indirect nature of the method, is the block design, alternating task blocks with blocks of passive rest Voxels showing a correlation with the seed region above a certain
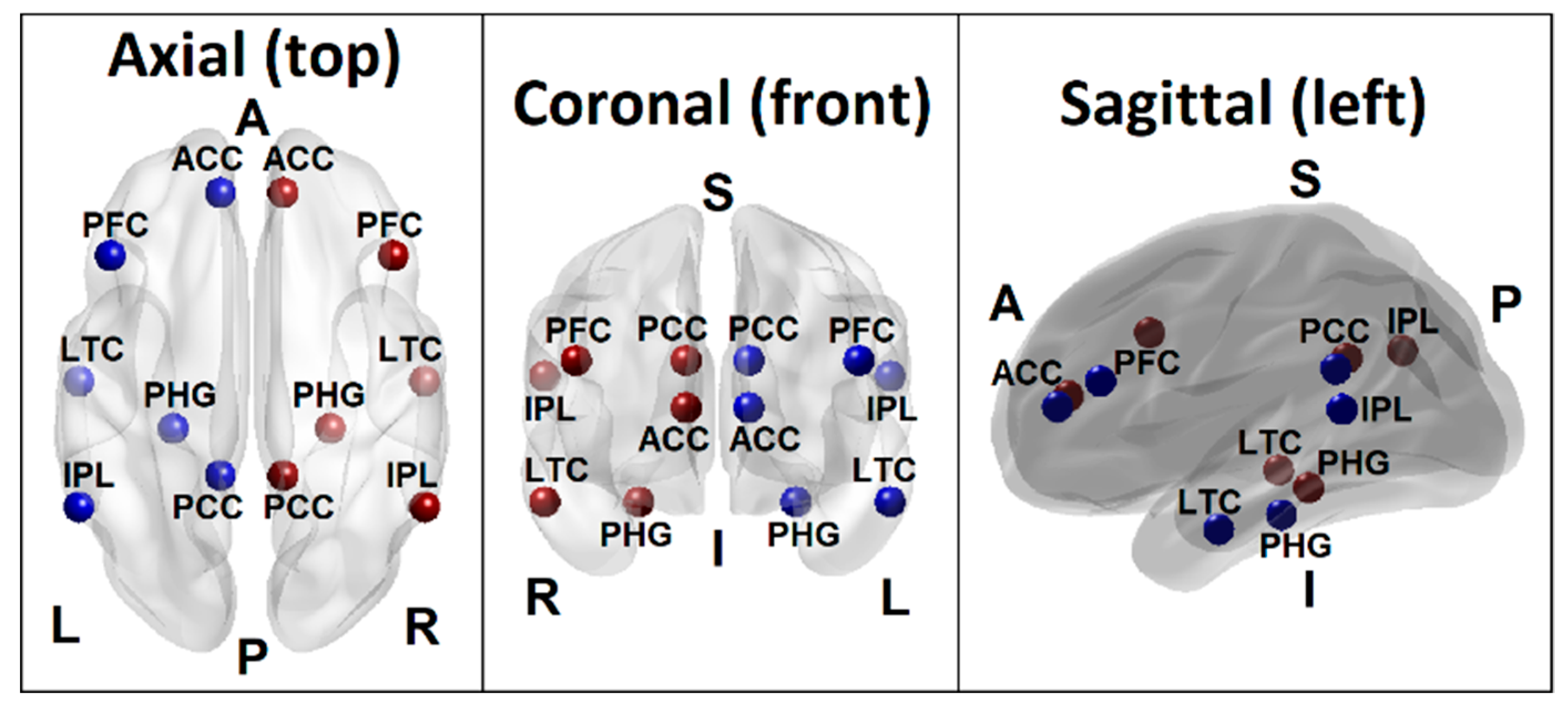



Behavioral Sciences Free Full Text Random Forest Classification Of Alcohol Use Disorder Using Eeg Source Functional Connectivity Neuropsychological Functioning And Impulsivity Measures Html
1 vector X ij and the outcome variables to be Y qij where q = 1, ,Q denotes the seed region (Y 1 ij) and its (Q − 1) regions of interest (ROIs), i = 1, , n denotes the ith subject, and j = 1, , J denotes the jth fMRI measurementSeedbased connectivity metrics characterize the connectivity patterns with a predefined seed or ROI (Region of Interest) These metrics are often used when researchers are interested in one, or a few, individual regions and would like to analyze in detail the connectivity patterns between these areas and the rest of the brainOr using multivariate methods, such
However, using the same rsfMRI data, with the seed region defined in the canonical counterpart of the Broca's area according to the standard coordinates, the seedbased FC mapping (Fig 4E,FResting state fMRI analysis using seed based and ICA methods Abstract In this paper, we analyze the functional connectivity between the various parts of the brain using resting state fMRI(Functional Magnetic Resonance Imaging)18 by American Journal of Neuroradiology
Similar to conventional taskrelated fMRI, the BOLD fMRI signal is measured throughout the experiment (panel a) Conventional taskdependent fMRI can be used to select a seed region of interest (panel b) To examine the level of functional connectivity between the selected seed voxel i and a second brain region j (for example a region in theOr using multivariate methods, such as independent component analysis (ICA)This correlation between timeseries of different regions of the brain Restingstate functional connectivity
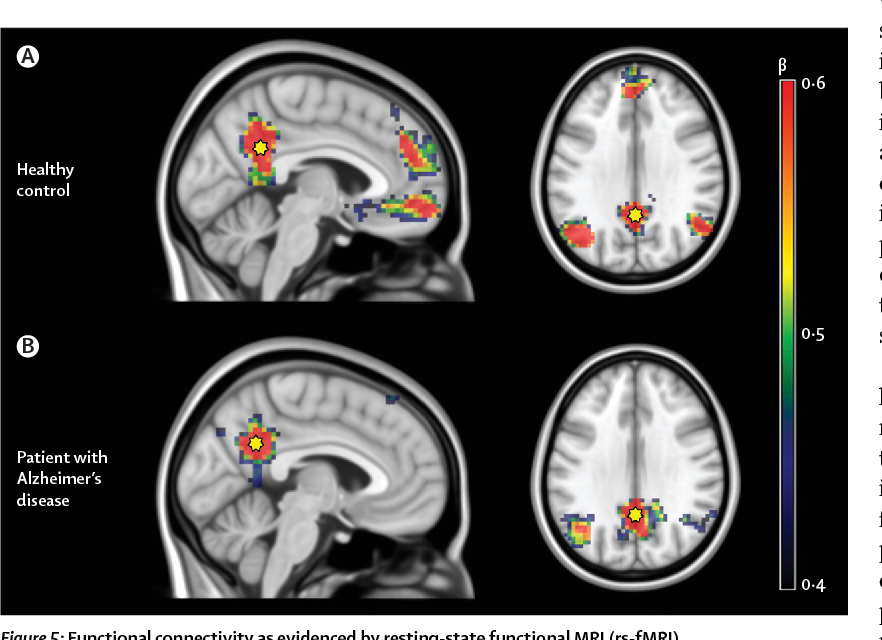



Figure 5 From Multimodal Imaging In Alzheimer S Disease Validity And Usefulness For Early Detection Semantic Scholar
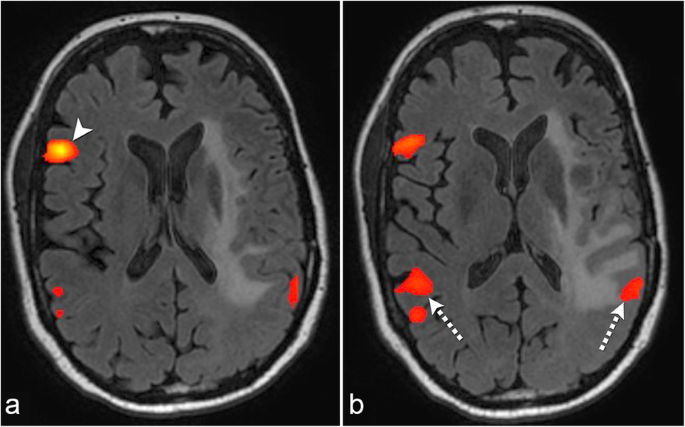



The Role Of Resting State Functional Mri For Clinical Preoperative Language Mapping Cancer Imaging Full Text
To better understand intrinsic brain connections in major depression, we used a neuroimaging technique that measures resting state functional connectivity using functional MRI (fMRI) Three different brain networks—the cognitive control network, default mode network, and affective network—were investigated Compared with controls, in depressed subjects each ofIn investigations of the brain's resting state using functional magnetic resonance imaging (fMRI), a seedbased approach is commonly used to identify brain regions that are functionally connected The seed is typically identified based on anatomical landmarks, coordinates, or the location of brain activity during a separate taskFMRI, during which the same subjects performed bilateral finger tapping After determining the correlation between the BOLD time course of the seed region and that of all other areas in the brain, the authors found that the left somatosensory cortex was highly correlated with homologous areas in the contralateral hemisphere




Seed Regions Of The Seed Based Resting State Analysis Seed Regions And Download Scientific Diagram
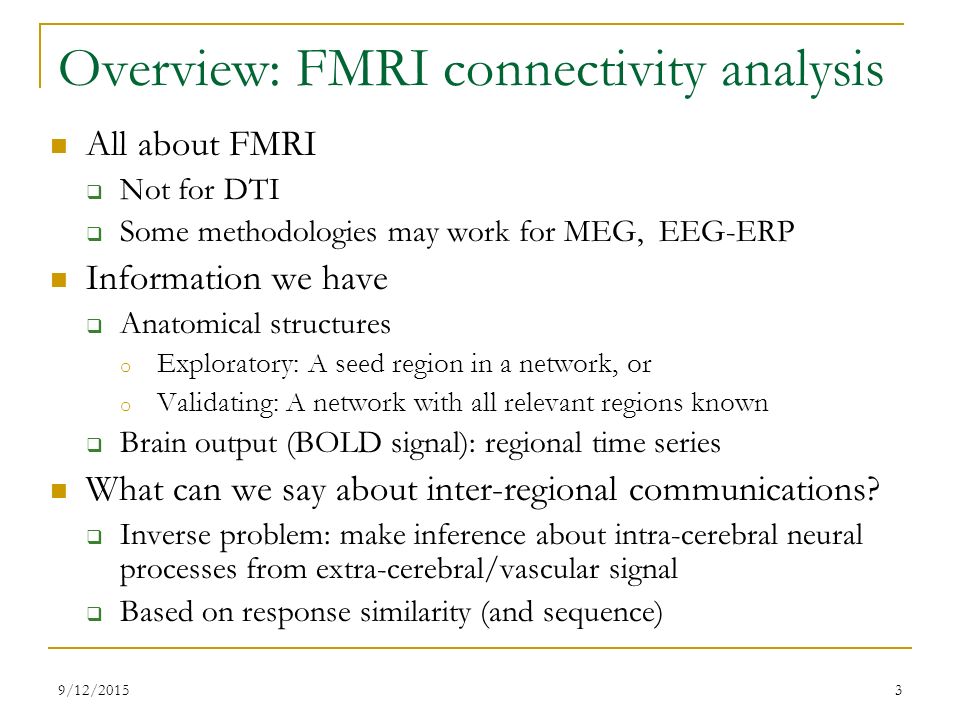



Fmri Connectivity Analysis In Afni Ppt Video Online Download
The map represents a restingstate functional connectivity analysis performed on 1,000 human subjects, with the seed placed at the currently selected location Thus, it displays brain regions that are coactivated across the restingstate fMRI time series with the seedAfter defining a seed mask in the Montreal Neurological Institute (MNI) standard space (Fig 1a), the time course of each voxel within the seed region was extracted from the rsfMRI datasets (Fig 1b), serving as the data matrix in sparse representation Importantly, both the wholebrain functional connectivity patterns and the local timevaryingFunctionally, the brain is subspecialized for perceptual and



Multimodal Neuroimaging Of Prosody Applied Social Neuroscience And Perception Laboratory



Onlinelibrary Wiley Com Doi Pdf 10 1002 Mrm
Summarize regions by average time course 2For each subject, calculate correlations between seed region and other regions of interest 3Transform Pearson correlations by Fisher's z;Recent research into restingstate functional magnetic resonance imaging (fMRI) has shown that the brain is very active during rest patterns can be identified based on how similar the time profile is to a seed region's time profile However, this method requires a seed region and can only identify one resting state network at a timeRestingstate fMRI The brain controls all the complex functions in the human body Structurally, the brain is organized grossly into different regions specialized for processing and relaying neural signals;




Does Resting State Functional Connectivity Differ Between Mild Cognitive Impairment And Early Alzheimer S Dementia Journal Of The Neurological Sciences
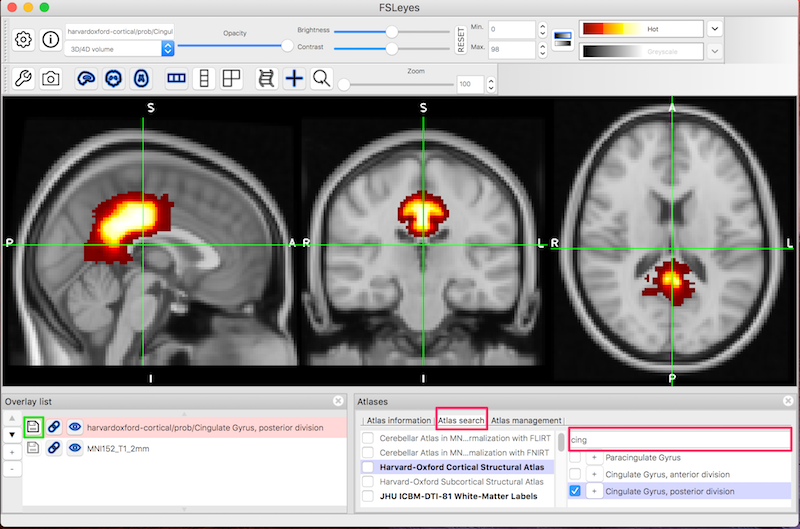



Fsl Fmri Resting State Seed Based Connectivity Neuroimaging Core 0 1 1 Documentation
The seed sequence is essential for the binding of the miRNA to the mRNA The seed sequence or seed region is a conserved heptametrical sequence which is mostly situated at positions 27 from the miRNA 5´end Even though base pairing of miRNA and its target mRNA does not match perfect, the "seed sequence" has to be perfectly complementaryFirst, however, we will need to create and place the seed region appropriately We can place a seed voxel in the vmPFC using the XYZ coordinates 0, 50, 5 (similar to MNI coordinates of 0, 50, 5), and a correlation coefficient willThe authors identified a seed region in the left somatosensory cortex on the basis of block design fMRI After determining the correlation between the BOLD time course of the seed region and that of all other areas in the brain, the authors found that the left somatosensory cortex was highly correlated with homologous areas in the contralateral hemisphere




Patient Tailored Connectivity Based Forecasts Of Spreading Brain Atrophy Sciencedirect



Resting State Functional Mri Everything That Nonexperts Have Always Wanted To Know American Journal Of Neuroradiology
0 件のコメント:
コメントを投稿